As the advantages of data-led modern transformation become more and more clear across industries, businesses today want to do more with data. It makes sense that interest in data tools, platforms, and innovative data management capabilities is rising, as well as the employability of data science talent, given that data is the lifeblood of innovation.
However, the proper infrastructure and strategy must be used to ensure success and scalability in order to supercharge innovation with data.
Like more money, we all desire more data. But if$ 100 billion was delivered to your home in change, would you know what to do?
From efficient storage to the computing power needed to process, analyze, and derive insights, managing data presents a number of challenges.
Furthermore, it is becoming more and more important to make sure that all of these components are provided within a cost-effective and lasting model because managing complex data infrastructure can quickly result in spiraling overhead costs and energy demands, particularly when scaling up is required.
utilizing the cloud’s power
Adopting the appropriate data platforms and data engineering tools is crucial for cutting costs and delivering efficiency because huge data projects at scale require a sizable amount of computational power.
For instance, the computing resources may need several hundreds of servers when analyzing terabytes of data. Adopting a more environmentally friendly strategy is necessary because managing this numerous servers together over several days will undoubtedly cause costs to skyrocket.
Any organization that needs to analyze large amounts of data will either need hundreds of on-site servers or use a virtualization model where servers are managed as services. This cloud-based model is more cost-effective and green in addition to providing flexibility and scalability.
For instance, it is possible to reduce the amount of administration and energy used for each workflow by spinning up servers as needed, for either production or development environments, and enable the flexibility to allocate resources where they are needed at any time.
Great data needs a ton of storage space. The scalability needed for safe, dependable, and limitless storage is also provided by cloud services. Cloud data centers give businesses the assurance that projects won’t come to an end if there is a problem at one site because backups will immediately take over in the event of failure.
Utilizing the strength of big cloud providers that have made significant investments in emissions-reduction technologies offers a way to meet ESG targets because data centers significantly contribute to global carbon emissions.
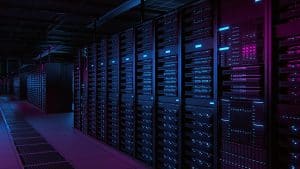
management of infrastructure
Numerous sources of data, each represented in a different format, will probably need to be integrated when working with large data in order to allow for accurate analysis. The time it takes to deliver results is greatly reduced by using tools like Terraform to create script and infrastructure templates that can be used with fresh data projects.
For instance, a script can be created to analyze satellite data collected in one area to understand industrial growth, and it can then be quickly applied to another area or geographical setting. The development of new indicators has been considerably accelerated, though there will undoubtedly be some tweaking, such as taking into account the particular factors and data availability across various geographies.
The same idea applies to completely different indicators as well. Much of the same workflow, i.e. template, can be applied to various indicators that use satellite data, such as one that focuses on deforestation, as in the example of an indicator that analyzes it for urban growth across a particular region.
This increases automation and enables businesses to quickly create innovative workflows and environments by analyzing comparable data sets across projects.
talent for data and expertise
Of course, talent is a crucial element in successfully completing significant data projects. Understanding the necessary data and validating the use case will depend on your subject matter expertise for each usage case.
For instance, a data project that deals with financial data should have its methodology checked and, in the best case scenario, built with the assistance of an economics expert. This is done to ensure that users, who may be paying for the insights provided, are not harmed by the methodology’s reliability.
Today’s large data projects also call for multidisciplinary data teams to handle both structured and unstructured data, which calls for a range of management skills. Delivering an innovative data project requires the ability to combine massive amounts of unstructured data with smaller sets of structured data, as well as the management skills for a data warehouse like SQL and an object storage data lake like Amazon S3.
These are the fundamental guidelines for delivering a data project at scale while optimizing every stage of development and delivery. Reducing rework and getting rid of infrastructure that isn’t up to par even has the added advantages of lowering operating costs and having a smaller negative impact on the environment.
For these reasons, it’s always worthwhile to consider areas where the infrastructure and processes mentioned in this article could be optimized to produce positive outcomes.